- Salle de réunion du 1e étage, LEM3 – site principal, 7 rue Félix Savart, 57070 Metz
Under the aegis of the Medicis programme
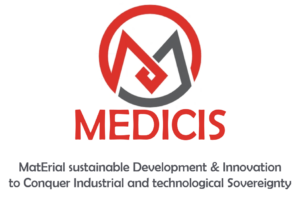
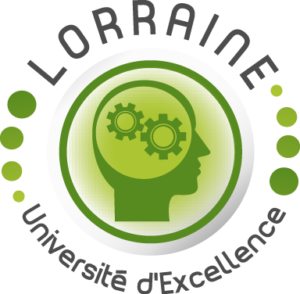
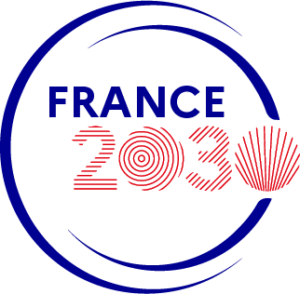
Resume
With the advent of machine learning (ML), the use of deep artificial neural networks (ANNs) for data-driven constitutive modeling has gained prominence in computational mechanics. This is particularly true in the context of numerical homogenization for heterogeneous materials. Nonetheless, ANNs are not without shortcomings for constitutive modeling. In their standard form, they are built to simply map input data to output data – typically, without fundamental restrictions. Thus, when such ANNs are exploited in physics-based numerical simulations, they can violate some laws of physics, in which case confidence in the simulation-based predictions is reduced. Hence, this lecture will present a trustworthy ML framework for the data-driven discovery of constitutive models for nonlinear elastic and viscoelastic heterogeneous materials that is broadly mechanics-informed. The framework enforces on the ANN’s architecture a long list of desirable mathematical properties that guarantees the satisfaction of an even longer list of physical/mechanical constraints, including: dynamic stability, material stability, and internal variable stability; objectivity; consistency; fading memory; recovery of elasticity; the second law of thermodynamics; and material non-inversion. The lecture will show that embedding these notions in a learning approach reduces a model’s sensitivity to noise and promotes its robustness to inputs outside the training domain. It will also highlight the merits of the proposed trustworthy ML framework for numerous engineering applications, including the prediction of the supersonic inflation dynamics of a parachute system with a canopy made of a woven fabric.
Biography
Charbel Farhat is the Vivian Church Hoff Professor of Aircraft Structures and the Director of the Stanford-KACST Center of Excellence for Aeronautics and Astronautics at Stanford University. He is a Member of the National Academy of Engineering (US); a Member of the Royal Academy of Engineering (UK); a Doctor Honoris Causa from Ecole Nationale Supérieure d’Arts et Métiers, Ecole Centrale de Nantes, and Ecole Normale Supérieure Paris-Saclay; a designated ISI Highly Cited Author in Engineering; and a Fellow of AIAA, ASME, IACM, SES, SIAM, USACM, and WIF. He has trained about 100 PhD and post-doctoral students. For his research on aeroelasticity, aeroacoustic scattering, CFD, dynamic data-driven systems, fluid-structure interaction, high performance computing, model reduction, and physics-based machine learning, he has received many professional and academic distinctions including: the Ashley Award for Aeroelasticity and the Structures, Structural Dynamics and Materials Award from AIAA; the Spirit of St Louis Medal and a Lifetime Achievement Award from ASME; the Gordon Bell Prize and the Sidney Fernbach Award from IEEE; the Gauss-Newton Medal from IACM; the Grand Prize from the Japan Society for Computational Engineering Science; the John von Neumann Medal from USACM; and the Olof B. Widlund Prize for Excellence in Domain Decomposition Methods from DDM.org. He served on the Scientific Advisory Board of the US Air Force and on the Space Technology Industry-Government-University Roundtable. He was also selected by the US Navy recruiters as a Primary Key-Influencer and flown by the Blue Angels.